Hi, I'm Nat! I work on frontier risk evaluations at
Scale AI, developing benchmarks to assess the dual-use capabilities of ML systems. Previously, I was an early researcher and technical writer at the
Center for AI Safety and studied computer science at UC Berkeley 🐻.
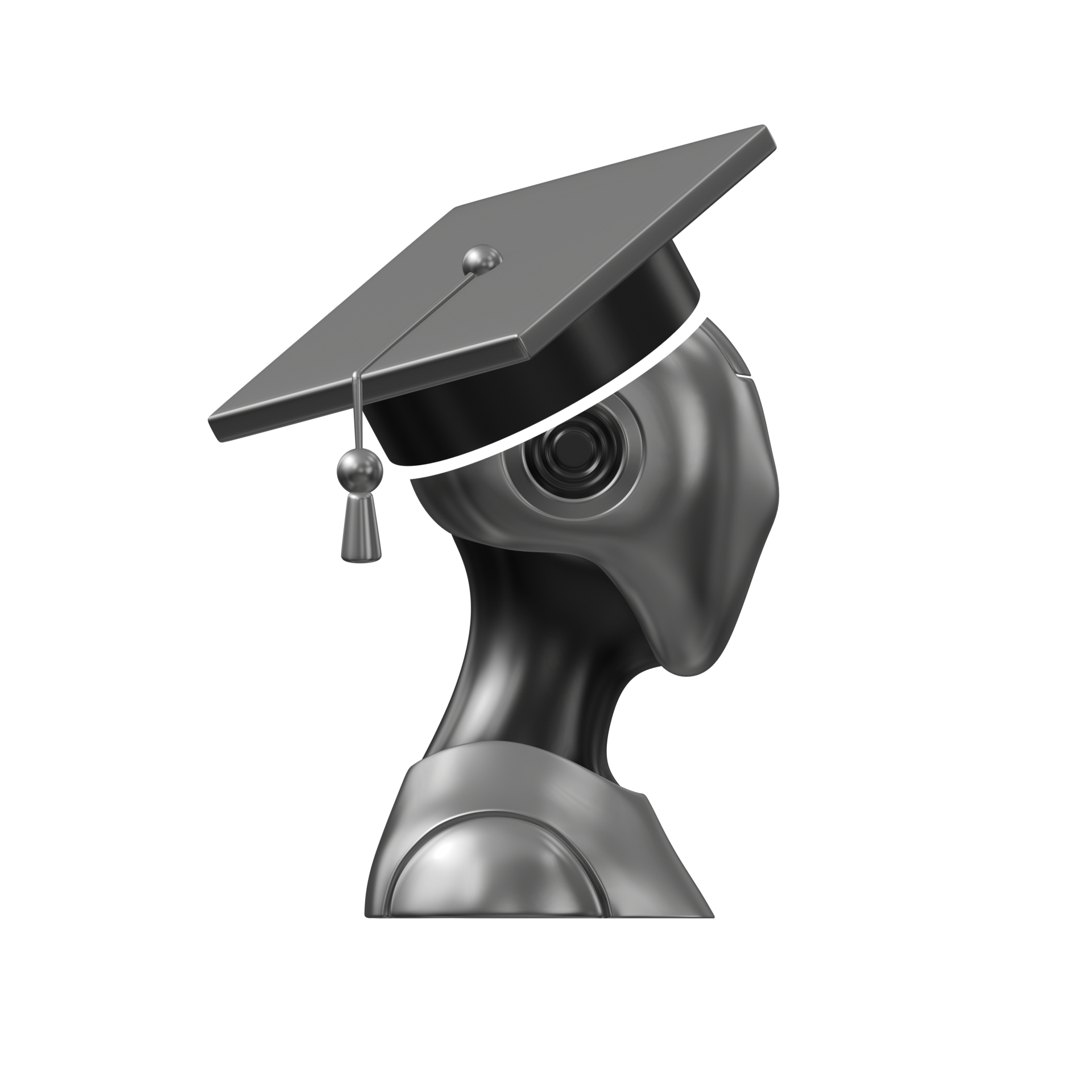
Humanity's Last Exam L. Phan*, A. Gatti*, Z. Han*, N. Li*, J. Hu, H. Zhang, A. Khoja, R. Kim, J. Hausenloy, O. Zhang, M. Mazeika, [633 not listed], S. Yue**, A. Wang**, D. Hendrycks** arXiv Preprint TL;DR ⬆️ / paper / website / dataset / New York Times Humanity's Last Exam (HLE) is an extremely challenging LLM benchmark consisting of 3,000 expert-designed questions spanning domains like mathematics, philosophy, and the sciences. HLE highlights a gap between AI and expert human capabilities, with state-of-the-art models achieving near-zero accuracy at the time of release.
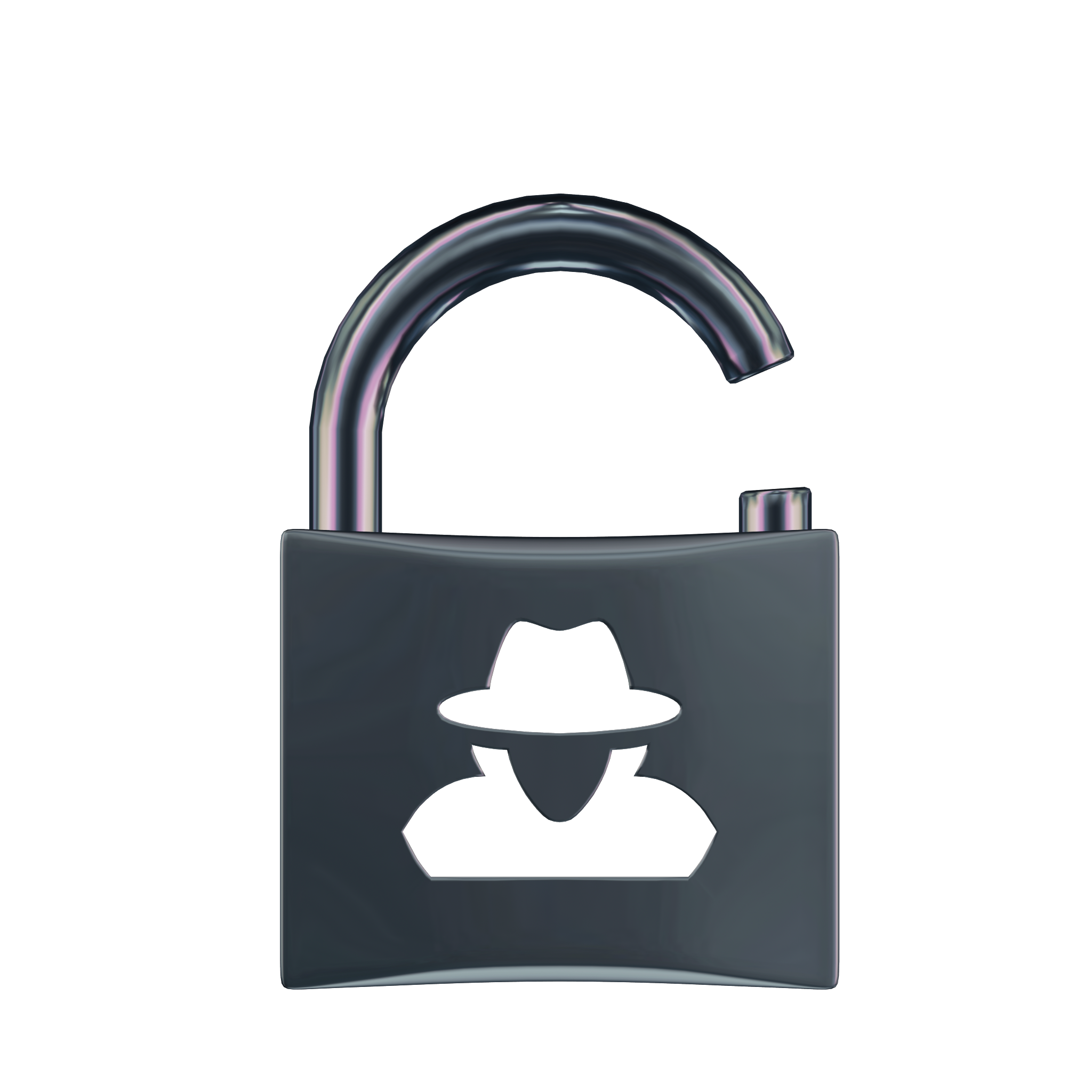
LLM Defenses Are Not Robust to Multi-Turn Human Jailbreaks Yet N. Li, Z. Han, I. Steneker, W. Primack, R. Goodside, H. Zhang, Z. Wang, C. Menghini, S. Yue NeurIPS 2024 Red Teaming Workshop (Oral) TL;DR ⬆️ / paper / website / dataset Current LLM defenses, which are remarkably robust against automated adversarial attacks, are not robust against humans who attack over multiple turns -- a more realistic threat model of malicious use in the real world``.

The WMDP Benchmark: Measuring and Reducing Malicious Use with Unlearning N. Li*, A. Pan*, A. Gopal†, S. Yue†, D. Berrios†, A. Gatti‡, J. Li‡, A. Dombrowski‡, S. Goel‡, L. Phan‡, G. Mukobi, N. Helm-Burger, R. Lababidi, L. Justen, A. Liu, M. Chen, I. Barrass, O. Zhang, X. Zhu, R. Tamirisa, B. Bharathi, A. Khoja, Z. Zhao, A. Herbert-Voss, C. Breuer, S. Marks, O. Patel, A. Zou, M. Mazeika, Z. Wang, P. Oswal, W. Lin, A. Hunt, J. Tienken-Harder, K. Shih, K. Talley, J. Guan, R. Kaplan, I. Steneker, D. Campbell, B. Jokubaitis, A. Levinson, J. Wang, W. Qian, K. Karmakar, S. Basart, S. Fitz, M. Levine, P. Kumaraguru, U. Tupakula, V. Varadharajan, Y. Shoshitaishvili, J. Ba, K. Esvelt, A. Wang**, D. Hendrycks** ICML 2024 TL;DR ⬆️ / paper / website / code / TIME / Scale AI blog / CAIS blog WMDP is a LLM benchmark surrounding hazardous knowledge in biosecurity, cybersecurity, and chemical security, for measuring and mitigating the risk of malicious use. Towards reducing risk, we introduce RMU, an unlearning method which reduces LLM performance on WMDP while retaining performance on general tasks.
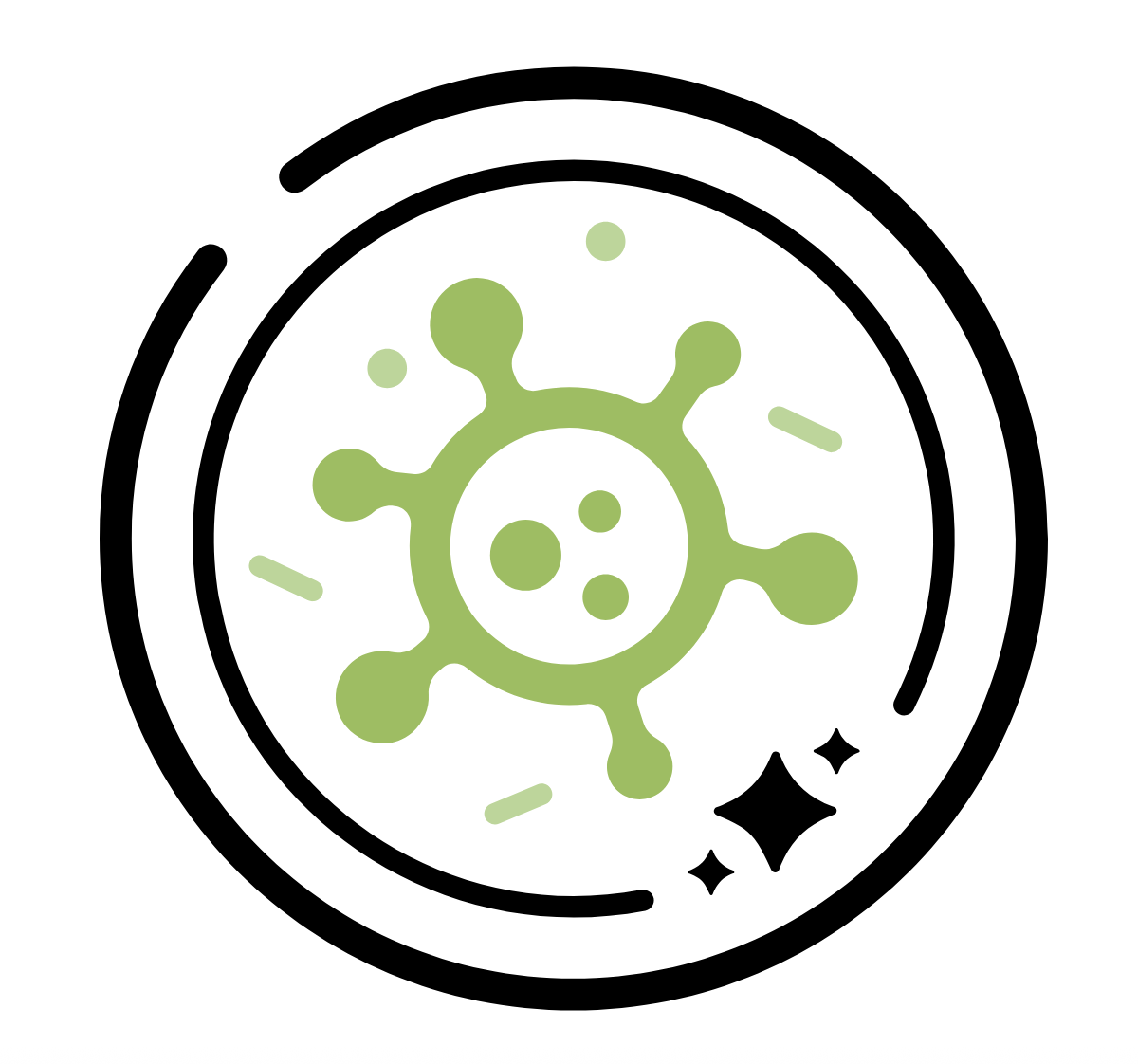
Virology Capabilities Test (VCT): A Multimodal Virology Q&A Benchmark J. Götting*, P. Medeiros*, J. Sanders*, N. Li, L. Phan, K. Elabd, L. Justen, D. Hendrycks, S. Donoughe arXiv Preprint TL;DR ⬆️ / paper / website / TIME VCT is an benchmark that measures LLMs' capabilities in troubleshooting complex virology lab protocols. It covers fundamental, tacit, and visual knowledge that is essential for practical work in virology laboratories. Frontier LLMs outperform expert virologists even within their sub-areas of specialization.
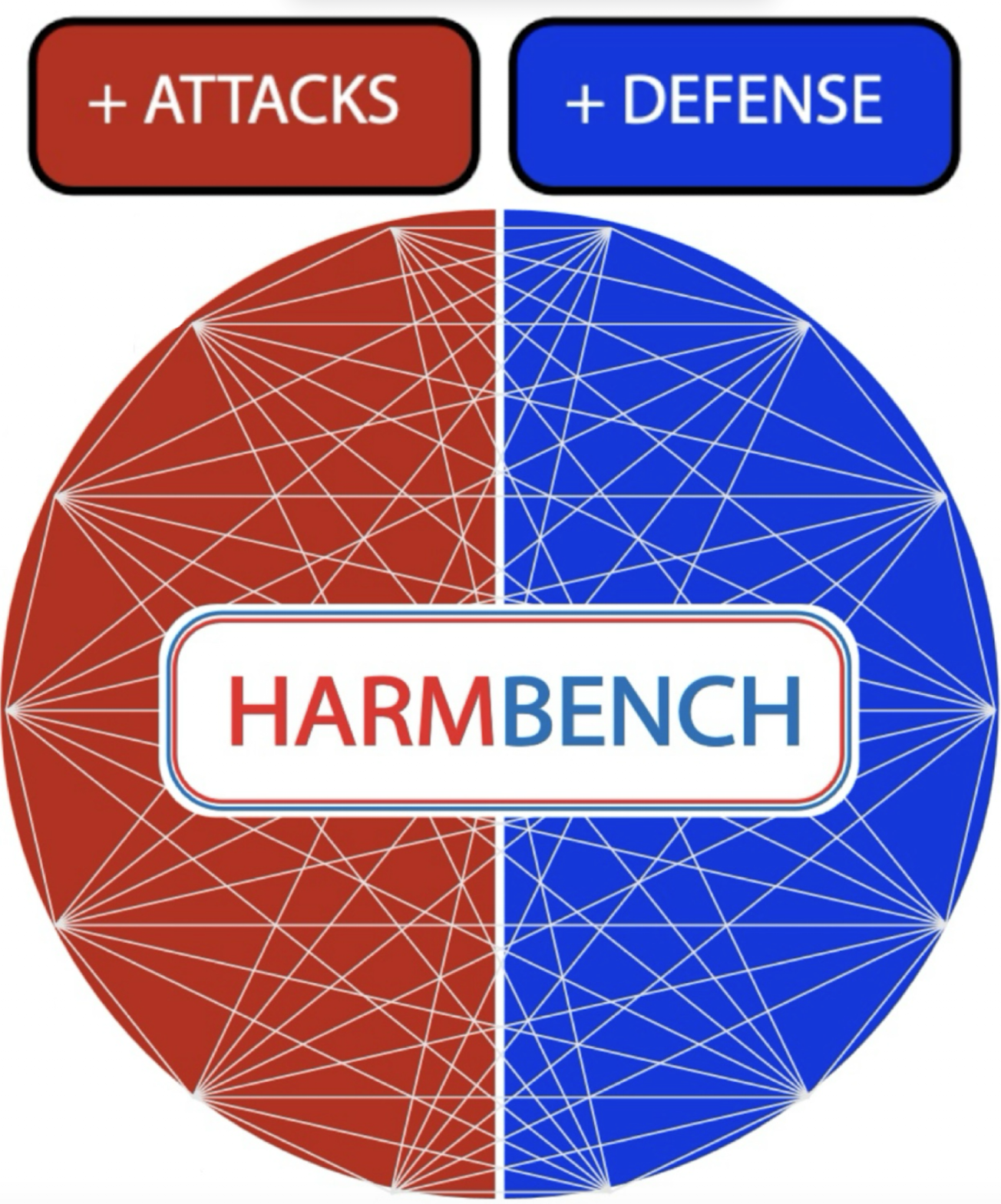
HarmBench: A Standardized Evaluation Framework for Automated Red Teaming and Robust Refusal M. Mazeika, L. Phan, X. Yin, A. Zou, Z. Wang, N. Mu, E. Sakhaee, N. Li, S. Basart, B. Li, D. Forsyth, D. Hendrycks ICML 2024 TL;DR ⬆️ / paper / website / code HarmBench is an evaluation framework for automated language model red teaming. We conduct a large-scale comparison of 18 red teaming methods and 33 target LLMs and defenses, proposing a highly efficient adversarial training method that enhances LLM robustness.
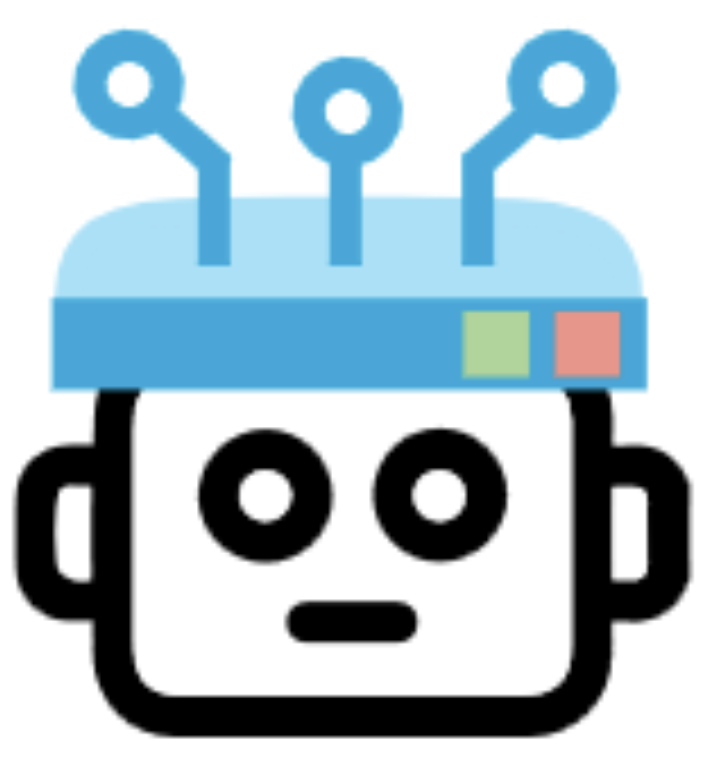
Representation Engineering: A Top-Down Approach to AI Transparency A. Zou, L. Phan*, S. Chen*, J. Campbell*, P. Guo*, R. Ren*, A. Pan, X. Yin, M. Mazeika, A. Dombrowski, S. Goel, N. Li, M. Byun, Z. Wang, A. Mallen, S. Basart, S. Koyejo, D. Song, M. Fredrikson, Z. Kolter, D. Hendrycks arXiv Preprint TL;DR ⬆️ / paper / website / code Representation engineering (RepE) enhances LLM transparency by monitoring and manipulating high-level cognitive phenomena. RepE is effective in mitigating dishonesty, hallucination, and other unsafe behaviors.
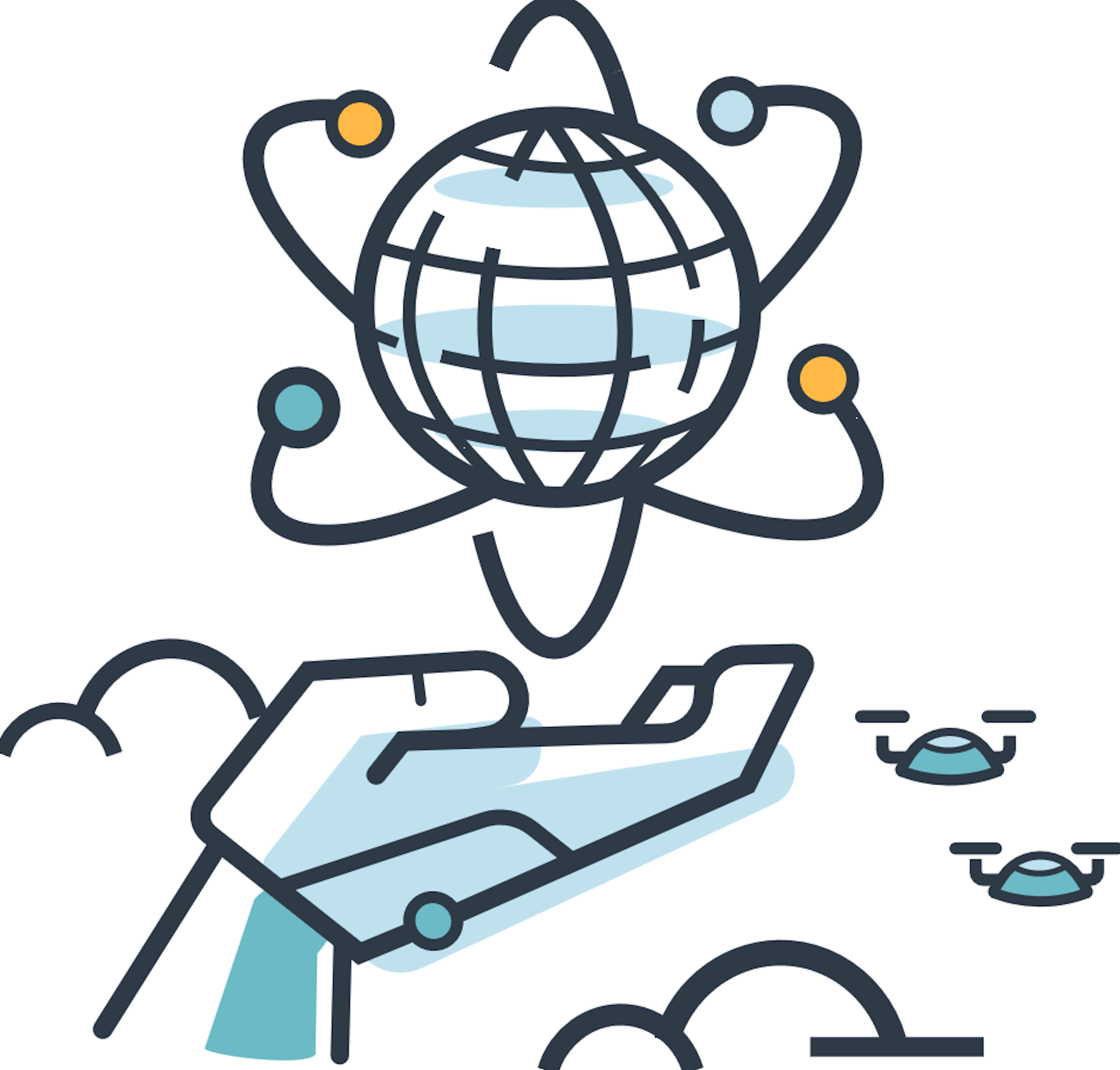
Do the Rewards Justify the Means? Measuring Trade-Offs Between Rewards and Ethical Behavior in the MACHIAVELLI Benchmark A. Pan*, C. Shern*, A. Zou*, N. Li, S. Basart, T. Woodside, J. Ng, H. Zhang, S. Emmons, D. Hendrycks ICML 2023 (Oral) TL;DR ⬆️ / paper / website / code MACHIAVELLI is a benchmark of 134 text-based choose-your-own-adventure games with annotations of safety-relevant concepts such as deception, physical harm, and power-seeking, guiding development towards safe yet capable language agents.
About
I’m forever grateful to be supervised by Dan Hendrycks and Summer Yue, and mentored by Alexander Pan, Cristina Menghini, Julian Michael, Steven Basart, and Zifan Wang. Outside of work, I’m a fan of overhang bouldering, legislative redistricting, playing by ear, Porter Robinson, and the United States 🇺🇸!